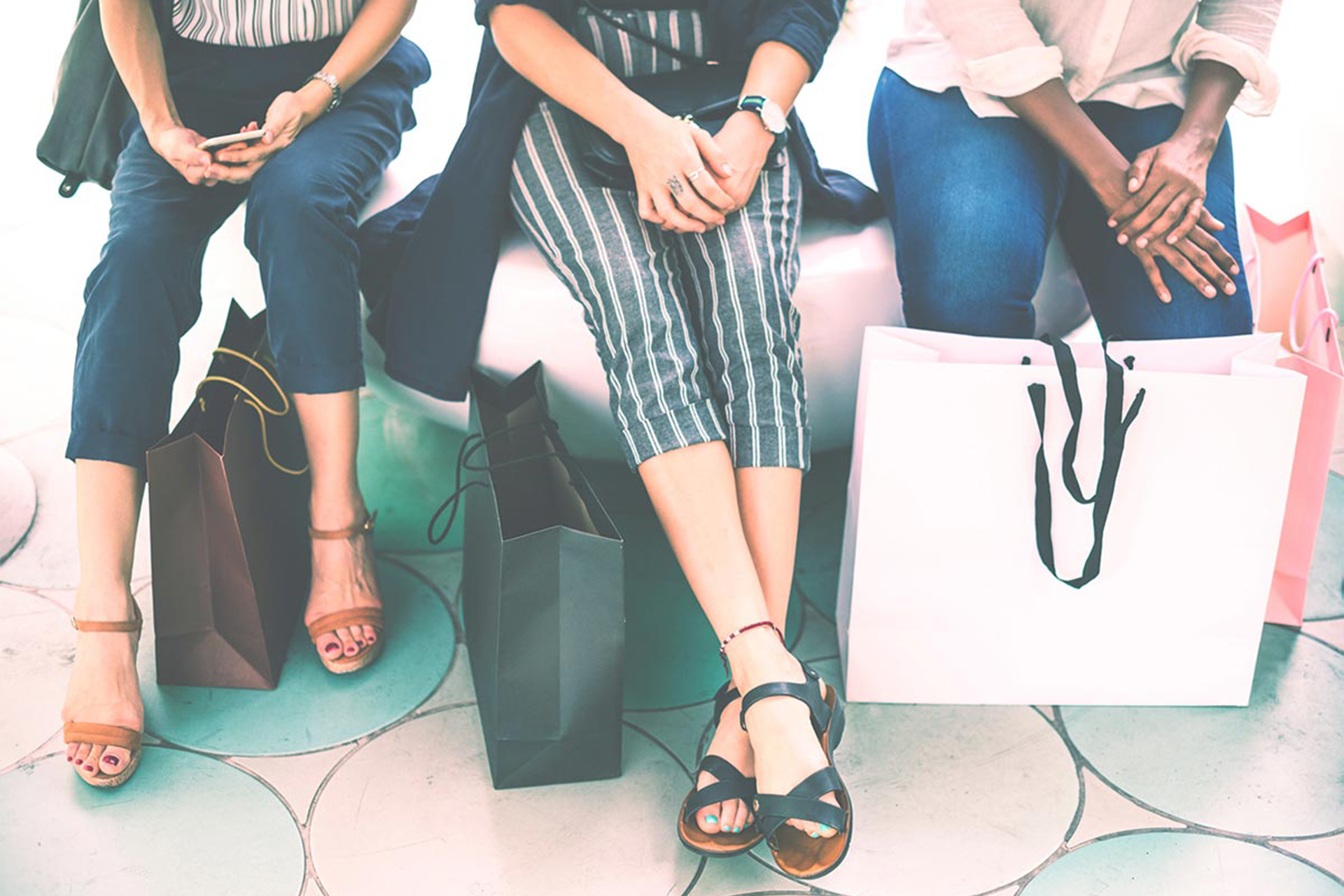
CLIENTELING : the ultimate customer knowledge
Clienteling is a long-standing practice born of local shops, where a notebook was filled with information gleaned from customers made it possible to make all the difference when making a purchase. Today, Clienteling is the ultimate brick of an omnichannel strategy.
But if the concept of “one to one” is well known, its application is enriched according to customer requirements and raises the following issue: what technical and business leverages should be activated to capitalize on 360° customer knowledge?
Clienteling, think business before tools
Clienteling aims to improve the customer experience not by multiplying gadget tools in the store, but rather by offering a high value-added relationship, fueled by customers and their data.
Personalization of interactions with the customer is the end as well as the means, and must be the result of a reflection at the initiative of the business:
|
![]() |
After having identified the target business vision, tools that can support this vision can be determined, because Clienteling is above all a marketing strategy with multiple operational variations. From the integration of appointment scheduling modules to the integration of flying checkouts or personalized shopping, the brands then use different levers to get to know the customer better.
Aligning marketing and technical issues is a real challenge because of the evolution of customer uses. In order to integrate increasingly qualified data, the Clienteling strategy must be thought in terms of short, medium and long-term deployment, supported by a scalable by design system.
Clienteling & Machine Learning, the ideal couple
Because there are no limits to customer knowledge (well, yes, hello RGPD), knowledge at instant T is not enough.
![]() |
One example is Burberry, which encourages customers to share their data with rewards through its loyalty program and now has a customer base of 12 million. By encouraging customers to provide data through its loyalty program, Burberry is providing itself with a quality customer reference base that is ready to be used.
By reconciling online and offline data, the brand can be proactive and offers high value-added services throughout the customer journey. |
Because beyond the “simple” product recommendations pushed on the sales host’s shelves, a Clienteling strategy backed by a Machine Learning solution makes it possible to completely rethink the omnichannel. By combining first, second and third-party data within an optimized DMP, brands can combine ultra-personalized recommendation engines, geofencing, online marketing and adapt additional products and services accordingly. This is a technical lever that is becoming more and more essential, if there is a strong synergy with the business.
Monitoring your Clienteling strategy, or how to reinvent classic Marketing KPIs
Calculating the return on business investment of the Clienteling strategy is a real challenge: how do I know if my Clienteling strategy is working? How can I isolate customer contact points in an omnichannel configuration? Driven by the business, Machine Learning answers these questions by promoting the emergence of KPIs representing the online/offline complementarity to follow customers throughout their journey. |
![]() |
Revenue trends can now be cross-referenced with conversion metrics, in-store customer retention, historical customer satisfaction… and feed into multi-level dashboards.
Burberry’s combination of in-store customer counting beacon and mobile campaigns monitoring has resulted in an 85% collection rate of customer data in PoS and a 50% improvement in its loyalty rate. This is a strong illustration of the growing impact of Machine Learning on the omnichannel performance of retailers. This trend is also confirmed by a survey by Statista (‘AI & Machine Learning use cases in the retail industry worldwide as of 2019’), according to which almost half of retailers have planned or already implemented a multi-channel strategy based on AI.
Finally, the scope of forecasting technology goes beyond marketing. Let’s mention Etam, which articulates its entire production chain around the Machine Learning to rationalize the financial and ecological costs related to unsold products. Or how the complementarity of IA and business lines allows to capitalize on product and customer data to optimize all operational layers.
You have a project?